“Explainable AI” (XAI) is a new market that encourages artificial intelligence software to be transparent and understood by people. Explainable AI, which is expected to reach 21 billion US in market size by 2030, has triggered large investments in startups and cloud giants as they compete to make AI software easier for humans (1).
The technology, which was first announced in July and was outlined in a LinkedIn blog post on Wednesday, is a significant step forward in allowing AI to “present its work” in a useful way (2).
LinkedIn, a subsidiary of Microsoft Corp., increased subscription revenue by 8% after equipping its sales force with AI software that forecasts clients who are likely to cancel and explains how it came to that decision.
While AI experts have no trouble building systems that generate precise predictions on a wide range of business outputs, they are learning that for those tools to be more useful to human users, the AI may have to explain itself through yet another algorithm.
The emerging field of Explainable AI, or XAI, has sparked significant investment in Silicon Valley as startups and cloud giants compete to make unreadable software more understandable. The debate in Washington and Brussels is also flaring, where regulatory authorities want to ensure automated decision-making is done fairly and transparently.
Artificial intelligence (AI) has the potential to reinforce societal biases such as those based on race, gender, and culture. And several researchers and experts believe that explanations are an essential aspect of reducing those detrimental impacts (3).
Moreover, as more businesses adopt AI and machine learning, knowing how algorithms arrive at certain outcomes will increase consumer trust and allow further AI applications.
Read Also: IP Protection, Copyright Issues With AI-Generated Work
Practical Value of Explainable AI
Over the last two years, consumer protection agencies in the United States, including the Federal Trade Commission, have emphasized that not explainable AI may be scrutinized. The EU may also pass the Artificial Intelligence Act, which includes the requirement that users be able to interpret computerized predictions next year.
Explainable AI supporters say it has improved the performance of AI applications in industries, including healthcare and sales. Google Cloud delivers explainable AI services that, for example, educate clients wanting to improve their systems on which pixels and training examples mattered most in detecting a photo’s subject.
However, skeptics argue that the explanations for why AI anticipated what it did are too shaky because the AI technology used to understand the machines is not up to par.
LinkedIn and others working on explainable AI agree that Each stage in the process – assessing predictions, generating explanations, testing their accuracy, and making them actionable for users – has potential for improvement.
At the same time, LinkedIn claims that its technology has generated actual value after two years of experimentation in a relatively low-stakes application. The 8% boost in renewal bookings beyond typical growth for the current financial year is confirmation of this. While LinkedIn did not provide a cash figure for the benefit, it called it significant.
Previously, LinkedIn salespeople depended on their judgment and periodic automated signals concerning clients’ service usage.
Now, AI can handle research and analysis with ease. LinkedIn’s CrystalCandle identifies previously ignored tendencies, and its logic aids salespeople in honing their techniques to retain at-risk clients on board and sell upgrades to others.
“It has helped seasoned salespeople by offering precise insights into how to navigate conversations with prospects. It has also aided new salespeople in getting started straight away,” LinkedIn’s director of machine learning and head of data science applied research, Parvez Ahammad (4), stated.
Read Also: AI in Marketing: Strategies, Examples, and Everything You Need to Know
But, Are These Explanations Even Necessary?
LinkedIn was the first to make predictions without explanations in 2020. A score of around 80% accuracy shows the possibility that a client who is set to renew will upgrade, stay the same, or cancel.
The salespeople were not completely convinced. When a client’s chances of not renewing were as slim as a coin toss, the team marketing LinkedIn’s Talent Solutions recruiting and hiring software was unsure how to adjust their strategy.
They started getting a short, auto-generated paragraph in July that highlighted the factors influencing the score.
For example, the AI determined that a client was likely to upgrade because the company had added 240 new employees in the previous year, and candidates had been 146 percent more responsive in the previous month.
In addition, an index that evaluates a client’s overall performance using LinkedIn recruiting tools increased by 25% in the last three months.
According to Lekha Doshi, LinkedIn’s vice president of global operations, sales representatives (5) now guide clients to training, support, and services that enhance their experience and keep them spending depending on the explanations.
Some AI scientists, however, wonder whether explanations are required. Searchers suggest they may harm by instilling a false sense of confidence in AI or causing design compromises that make forecasts less accurate.
According to Fei-Fei Li, co-director of Stanford University’s Institute for Human-Centered Artificial Intelligence (6), people utilize goods like Tylenol and Google Maps. The inner workings of these products are not well known. Yet, extensive testing and observation have eliminated the majority of reservations about their effectiveness.
Similarly, even if individual decisions are incomprehensible, AI systems could be considered fair, said Daniel Roy, an associate professor of statistics at the University of Toronto (7).
According to LinkedIn, an algorithm’s integrity could not be assessed without understanding how it works.
It also claims that its CrystalCandle tool could aid AI users in other fields. Doctors may discover why AI thinks a person is more likely to develop a disease, and people could learn why AI suggested they be denied credit.
Been Kim, an AI researcher at Google (8), explained that the objective is that explanations will indicate whether a system matches the ideals and values that one wants to promote.
“I see interpretability as allowing machines and people to have a conversation,” she remarked.
Read Also: AI Will Disrupt the Content Marketing Space in 2022 & Beyond
Explainable AI and Shared Interest
While explainable AI exists to help experts make sense of a machine’s reasoning, these systems frequently only supply information on a single option at a time and require manual review. Models are frequently trained using millions of data inputs, making it nearly hard for humans to analyze them thoroughly enough to find patterns.
MIT and IBM Research researchers have developed a mechanism that allows users to aggregate, classify, and rank these unique explanations to examine a machine-learning model’s behavior quickly. Their method, known as Shared Interest, uses quantitative indicators to assess how well a model’s thinking resembles a human’s (9).
Shared Interest could make it simple for a user to notice disconcerting trends in a model’s evaluation instance; the model may be readily confused by irrelevant, redundant features such as background objects in images. By combining these insights, the operator might rapidly and quantitatively decide whether a model is reliable and ready to be implemented in a real-world setting.
“Our goal in developing Shared Interest was to be able to boost this analysis process so that you could recognize your model’s behavior on a more global level,” says lead author Angie Boggust, a grad student in the Computer Science and Artificial Intelligence Laboratory’s Visualization Group (CSAIL) (10).
Boggust collaborated on the study with her advisor, Arvind Satyanarayan, a computer science assistant professor who directs the Visualization Group (11) and IBM Research’s Benjamin Hoover (12), and senior author Hendrik Strobelt (13). They will present the work at the Conference on Human Factors in Computing Systems.
Shared Interest uses saliency approaches, which are common strategies for demonstrating how a machine-learning model made a certain conclusion. Suppose the model is classifying images; in that case, the saliency method highlights portions of an image relevant to the model when it reaches its judgment when the model was classifying images. A saliency map is a heatmap commonly superimposed on the source image to visualize these areas.
For instance, if the model identified the image as containing a dog and emphasized the dog’s head, the model considered those pixels essential when determining the image’s dog content.
Saliency approaches are compared to ground-truth data in Shared Interest. Ground-truth data are often human-generated annotations that encircle the pertinent areas of each image in an image dataset. The box would have surrounded the entire dog in the preceding scenario.
Shared Interest contrasts the model-generated saliency data with the human-generated ground-truth data for the same image when testing an image classification model to evaluate how well they align.
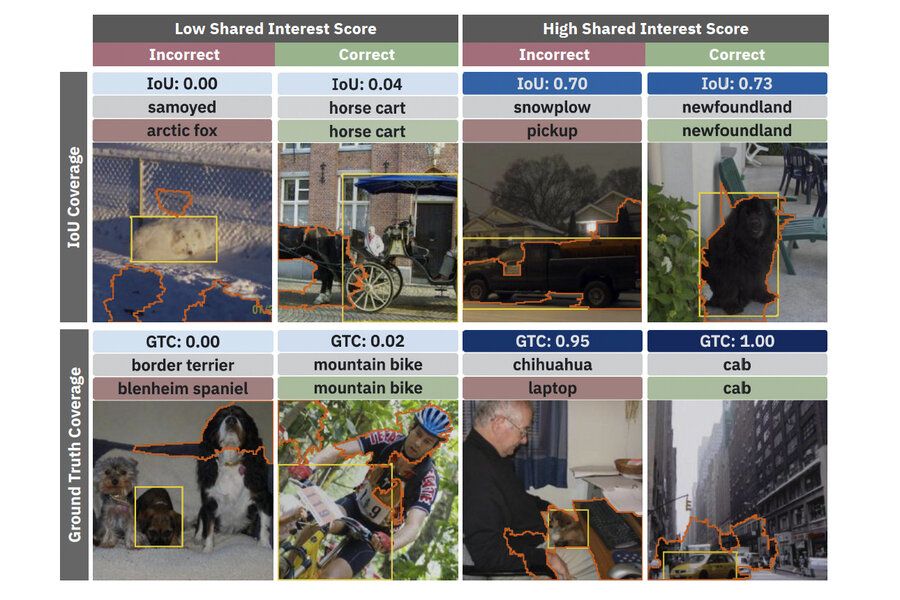
The method quantifies the alignment (or misalignment) using numerous indicators before categorizing a decision into one of eight categories. The categories range from perfectly human-aligned (the model makes a correct prediction, and the highlighted area in the saliency map is equivalent to the human-generated box) to completely distracted (the model makes an accurate prediction and the highlighted area in the saliency map is identical to the human-generated box) (the model makes an inaccurate prediction and does not use any image features found in the human-generated box).
The method highlights keywords rather than image regions when working with text-based data.
The researchers were impressed with Shared Interest’s performance in these case studies. However, Boggust emphasizes that the technique is only as good as the saliency methodologies upon which it is built. Shared Interest will acquire the limitations of those strategies if they carry bias or are erroneous.
The researchers hope to apply Shared Interest to many forms of data in the future, including tabular data seen in medical records. They also seek to employ Shared Interest to aid in the improvement of current saliency strategies.
Boggust thinks this study will spur greater research into explainable AI and quantifying machine-learning model behavior in understandable ways to humans.
Read Also: Cybersecurity Industry Could See a Jump in Investments with Google’s Latest Acquisition
AI Vs. Human
LinkedIn, a Microsoft subsidiary, began using XAI software in July and has already seen an increase of 8% in sales revenue. CrystalCandle, a LinkedIn application, identifies hidden trends and provides data to salespeople to retain at-risk customers on board and push others on upgrades. Transparent AI algorithms could help with more than just increased sales.
Regulators in the financial services industry, for example, seek to guarantee that machine learning is implemented in products and services, and approval/denial decisions are not discriminatory. With trillions of dollars on the line, it will become inevitable for algorithms to justify their decisions as AI becomes more integrated into banking. With improvements, the financial services industry might increase its AI use from 30% to a significant 50% by 2024 (14, 15).
As AI advances, researchers anticipate it will be able to prevent approximately 86 percent of diagnosis, prescriptions, and treatment errors (16).
Read Also: Biohacking: An Industry With Opportunities Worth Over $50 Billion
Closing Remarks
According to Research and Markets, the explainable AI (XAI) market worldwide will rise from 3.50 billion USD in 2020 to 21.03 billion USD in 2030 (17).
“In artificial intelligence, explainable AI is a technique in which the result can be evaluated and understood by humans. It differs from standard machine learning techniques, in which engineers frequently fail to grasp why the algorithm has reached a particular conclusion,” says Research and Markets.
Artificial intelligence is anticipated to boost global GDP by 1.2% per year. China (26% GDP boost) and North America (14.5% GDP boost) will reap the most economic benefits from AI, totaling $10.7 trillion and accounting for roughly 70% of the worldwide economic impact (18).
With a high-stakes future ahead, developers may soon be obliged to use transparent, explainable AI in their algorithms to generate confidence, either by law, market demand, or both. This increased transparency may inspire more diversified AI uses in hitherto non-technical fields.
Understandable and transparent artificial intelligence will be required for everything from financial decisions to medical diagnostics to autonomous vehicle systems.